Publication Details
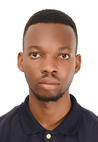
JOEL DZIDZORVI KWAME DISU
- NUGS-Shanghai
- Biomedical Engineering (Masters)
- Shanghai Jiao Tong University
2020 IEEE International Conference on Industry 4.0, Artificial Intelligence, and Communications Technology (IAICT)
22 Aug 2020 | 09:45
The main objective paper is to make an empirical analysis of the effect of various unstated spatial goal constraints on reinforcement learning policy for the “reacher†task in the Robotic Scrub Nurse (RSN) application. This “reacher†task is an essential part of the RSN manipulation task, such as the task of picking, grasping, or placing the surgical instruments. This paper provides our experimental results and the evaluation of the “reacher†task under different spatial goal constraints. We researched the effect of this unstated assumption on a reinforcement learning (RL) algorithm: Soft-Actor Critic with Hindsight Experience Replay (SAC+HER). We used the 7-DoF robotic arm to evaluate this state-of-the-art deep RL algorithm. We performed our experiments in a virtual environment while training the robotic arm to reach the random target points. The implementation of this RL algorithm showed a robust performance, which is measured by reward values and success rates. We observed, these reinforcement learning assumptions, particularly the unstated spatial goal constraints, can affect the performance of the RL agent. The important aspect of the “reacher†task and the development of reinforcement learning applications in medical robotics is one of the main motivations behind this research objective.