Publication Details
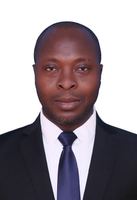
ISAAC KWESI NOONI
- NUGS-Nanjing
- Photogrammetry & Remote Sensing (Phd)
- Nanjing University Of Information Science And Technology
Pharmacognostic Evaluation and Physicochemical Analysis of Paullinia pinnata L. (Sapindaceae) 06 Feb 2020
Journal of Pharmacognosy and Phytochemistry
Quantile Mapping Bias Correction on Rossby Centre Regional Climate Models for Precipitation Analysis over Kenya, East Africa 06 Feb 2020
Preprints
The heavy metal contents of some selected medicinal plants sampled from different geographical locations 06 Feb 2020
Pharmacognosy Research Journal
Evaluation of the Rossby Centre Regional Climate Model Rainfall Simulations over West Africa Using Large-Scale Spatial and Temporal Statistical Metric 06 Feb 2020
Atmosphere
High Spatial Resolution Simulation of Sunshine Duration over the Complex Terrain of Ghana 06 Feb 2020
Sensors
Assessing contract management as a strategic tool for achieving quality of work in Ghanaian construction industry: A case study of FPMU and MMDAs 06 Feb 2020
Journal of Financial Management of Property and Construction
Evapotranspiration and its Components in the Nile River Basin Based on Long-Term Satellite Assimilation Product 06 Feb 2020
Water
International Journal of Remote Sensing
06 Feb 2020 | 20:19
Support vector machines (SVMs) have been frequently shown to result in more accurate classification than other image classification methods. However, few studies have successfully quantified their performance for mapping oil palm plantations. Various sustainability criteria developed by the Round Table on Sustainable Palm Oil (RSPO) have a spatial component but they provide little guidance on mapping oil-palm-related cover changes. SVM and maximum likelihood classifier (MLC) classification approaches in classifying oil palm plantations with Landsat ETM+ were compared. The best combination of three bands from the satellite image was selected based on Bhattacharyya distance. SVM and MLC performance was evaluated using overall accuracy assessment and kappa statistics. Bands 4, 5, and 3 provided the best spectral separability indices based on Bhattacharyya distance. SVM classification resulted in an overall accuracy of 78.3% (kappa statistic 0.73) compared with MLC, with an overall accuracy of 71.9% (kappa statistic 0.65). The performance of the SVM method is mainly affected by the accurate setting of parameters involved in the algorithm. The radial basis function parameter setting in SVM was an important variable in the classification process, and SVM improved the classification of oil palm mapping. Although the classification accuracy is still insufficient for large-scale implementation of the technique, further refinements may provide a way forward towards producing baseline information useful for RSPO certification.